DIFFUE: Towards More Robust Unlearnable Examples Leveraging Diffusion Models
Jan 1, 2024·
,,·
0 min read
Irfan Meerza

Oktay Ozturk
Amir Sadovnik
Jian Liu
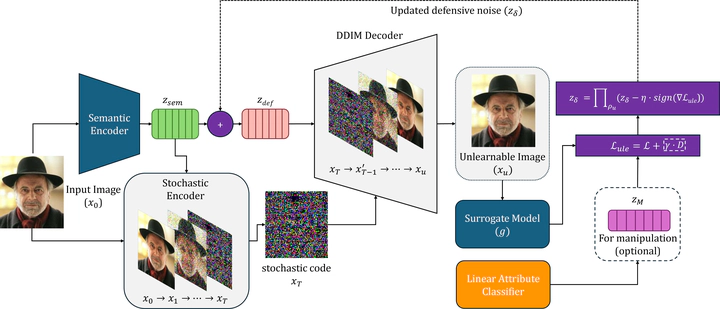
Abstract
The widespread sharing of images on social media and public platforms poses significant risks, as unauthorized third parties can scrape these images without permission and misuse them for AI training, often for commercial or even malicious purposes, leading to violations like unauthorized facial recognition or targeted advertising. To mitigate this issue, the concept of ‘unlearnable examples’ was developed, introducing imperceptible noise to images to degrade the generalizability of models attempting to learn from them, effectively disrupting unauthorized usage and safeguarding data integrity and individual rights. However, existing methods that add noise in the pixel space are susceptible to simple transformations and adversarial training, which can effectively bypass the defensive noise. Additionally, additive noise often comes at the cost of significant image quality degradation, leading to a trade-off between unlearnability and usability. In this paper, we introduce DiffUE to address these limitations by adding noise to the semantic space of images rather than the pixel space. This method preserves both image quality and usability while ensuring robust unlearnability, even against adversarial training, advanced relearning strategies, and lossy image compression, etc. Moreover, by targeting the semantic space, we can make purposeful modifications to the image, as opposed to the random noise typically added in other methods. Extensive experiments on three datasets, CIFAR-10, CelebA-HQ, and ImageNet, demonstrate that DiffUE achieves superior unlearnability while preserving higher image quality, providing a more robust and effective solution for safeguarding personal data in an increasingly exploitative AI landscape.
Type
Publication
Proceedings of the IEEE/CVF Conference on Computer Vision and Pattern Recognition (CVPR)